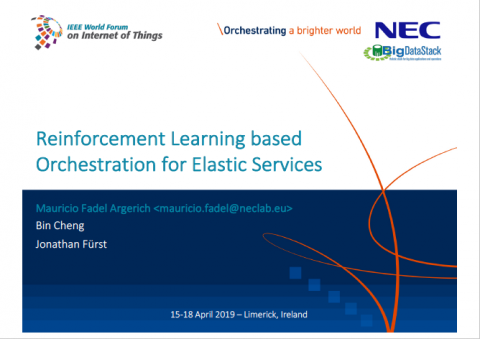
Increased data traffic and network utilization are one of the biggest challenges for network operators nowadays. One of the reasons is the massive amount of data generated by devices in the edge in the context of the Internet of Things (IoT). Edge computing allows network operators to reduce network stress and improve service responsiveness by allocating computation closer to data producers and consumers. Nonetheless, edge processing hardware is constrained and heterogeneous, which makes it hard to provide cloud-like elasticity features (i.e., scale out). For example, the load of a local edge server that serves an augmented reality (AR) application is directly correlated to the number of active users. Too many active users result in exhaustive response times and poor user experience.
Due to the highly variable execution context in which edge services run, adapting their behavior to the execution context is crucial to comply with their requirements. However, adapting service behavior is a challenging task because it is hard to anticipate the execution contexts in which it will be deployed, as well as assessing the impact that each behavior change will produce.
In order to provide this adaptation efficiently, we propose a Reinforcement Learning (RL) based Orchestration for Elastic Services. We implement and evaluate this approach by adapting an elastic service in different simulated execution contexts and comparing its performance to a Heuristics based approach. We show that elastic services achieve high precision and requirement satisfaction rates while creating an overhead of less than 0.5% to the overall service.
In particular, the RL approach proves to be more efficient than its rule-based counterpart; yielding a 10 to 25% higher precision while being 25% less computationally expensive.