Smart Insurance
Insurance companies increasingly need IT data-based solutions in order to address their needs about the provision of services according to the customer “tailored” requirements. The challenge is to allow insurance companies to better develop the customer management, by providing personalized services to the customer, as well as new corporate services for the handling of the customers’ profitability. A multi-channel scenario will be developed by GFT which will facilitate data analytics-powered smart insurance, providing a 360-degree view of the customer and personalized services. GFT will collaborate with HDI Assicurazioni, part of the Talanx Group of Hannover, for its adoption.
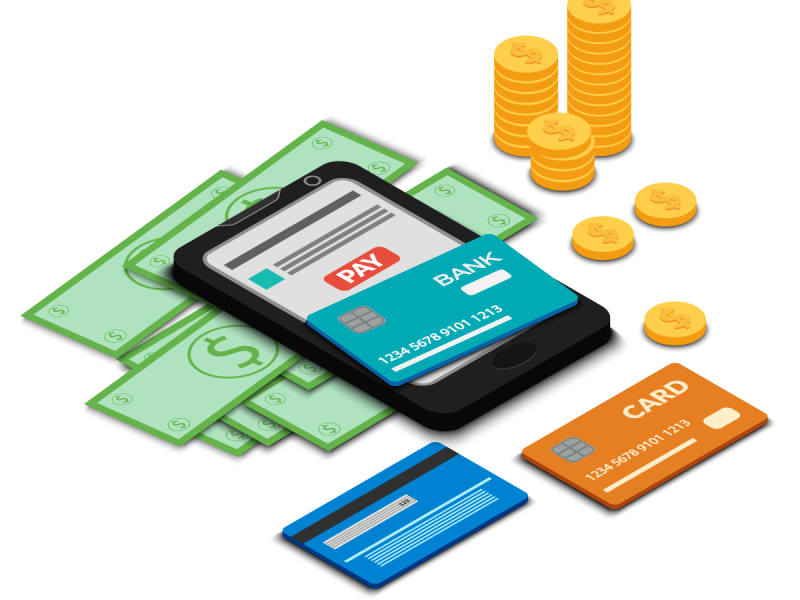
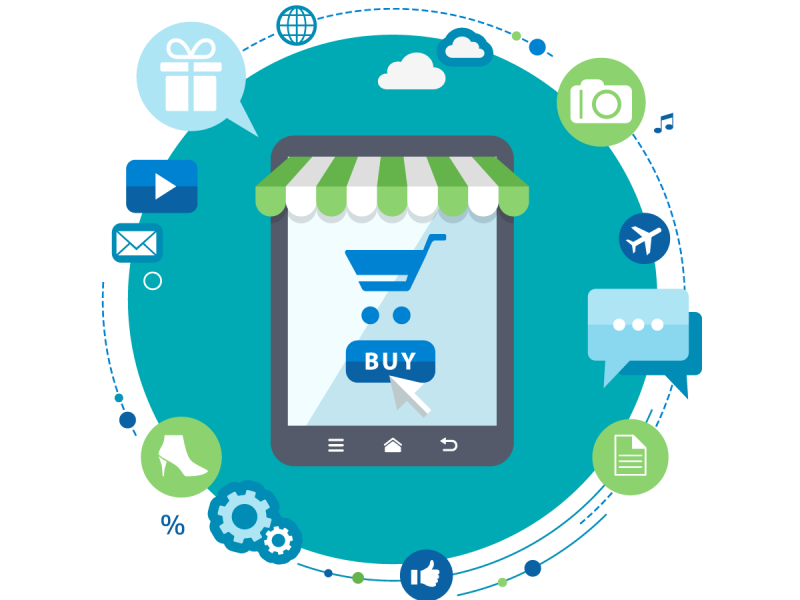
The Connected Consumer
This use case application will provide retailers with optimal insights into consumer preferences and improve the effectiveness of marketing strategies for improving consumer shopping experience.
This will be used by ATOS, which is currently defining a roadmap for a major Spanish food retailer that will allow them to offer predictive shopping lists, and tailored recommendations and promotions.
Real-time Ship Management
The BigDataStack algorithms will optimize and help cut costs on maintenance and spare parts inventory planning and dynamic routing.
These predictions will be estimated and provided to DANAOS, a leading international maritime player with more than 60 container ships.
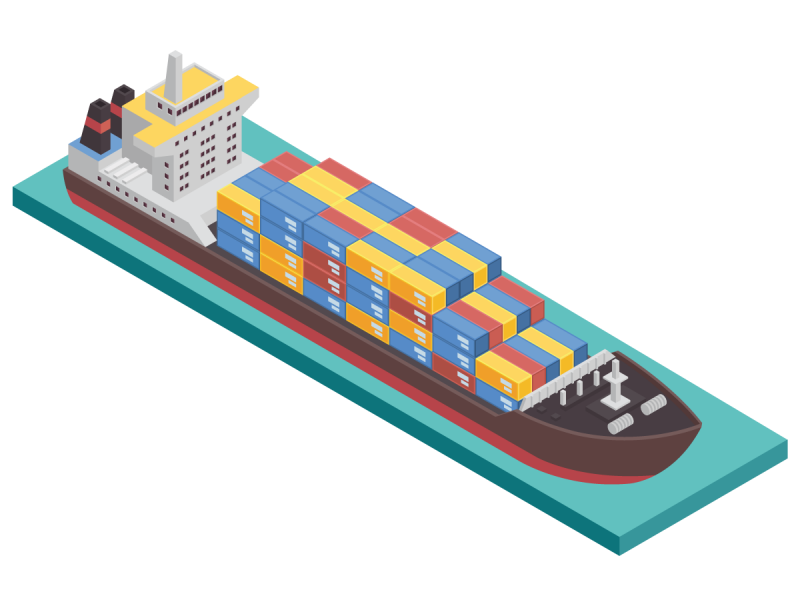