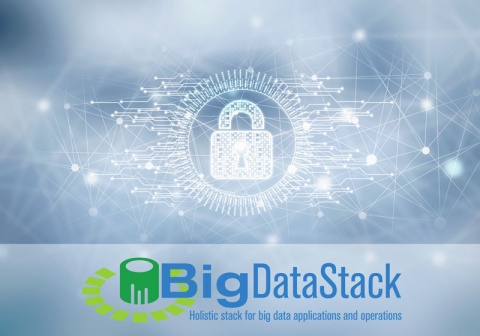
Big data in Europe for 2020 and beyond: Policy insights and recommendations from current H2020 big data projects.
This policy brief reflects current developments within the several Big Data research projects funded under H2020 and, combined with insights from the BDV PPP summit in Riga, aims to contribute to ongoing challenges in Europe around the regulation of big data. This policy brief is a product of the Common Dissemination Booster, funded under H2020. The policy recommendations are based on projects participating in the CDB services.
One of the main challenges identified in this policy brief is that of regulating big data. The contributors represent a multidisciplinary set of scholars, researchers and practitioners involved in either implementing big data solutions, researching data policy and governance, or finding technological solutions for implementing data policies. These activities are distinctly different, yet are intrinsically connected through questions of how Europe can maximize big data benefits while simultaneously protecting the rights of individuals and companies.
In the policy brief, we draw from a set of insights and lessons learnt that are based on recent H2020 projects around bigdata development and implementation in different sectors, ranging from traffic and transport to online retail to the public sector and more. The main solutions offered from the projects are (among others) a data governance taxonomy, tools for automated compliance, a Data Asset Marketplace and a roadmap for using big data for policymaking.
The main recommendations are to support integration and interoperability of public administration databases; to support the development of data markets and provide guidance on their effective use, to support work on the adoption of privacy-preserving technologies for big data and AI and to promote data-driven policymaking and regulatory automation.
Insights and recommendations from BigDataStack on Lowering barriers for the adoption of big data analytics - by Mauricio Fadel (NEC)
Nowadays, news about AI and big data analytics being successfully implemented in very different areas such as transportation, health and retail have become the norm. These AI and bigdata applications offer valuable insights and projections for decision making as well as automatizing a great part of business processes and bringing benefits to several fields. However, it is important to note that all of these great achievements are not breakthroughs in the science of AI or big data, but are implementations of these techniques to different use cases. These implementations are possible thanks to knowledge breakthroughs and technological advances that have already happened mostly more than a decade ago. This means that there is a huge opportunity for all organizations to benefit from AI and big data; because the knowledge is already out there and needs engineering and implementation efforts. For an organization that successfully implements AI and big data analytics, these benefits include reducing costs, providing useful insights and increasing productivity.
However, this adoption is not an easy task and has not always been successful due to the big challenges big data presents. To use big data, organizations must define and properly implement multiple processes, overcoming technical and legal challenges.
How BigDataStack is lowering the barriers for adoption of BigData Analytics
BigDataStack is developing a platform that offers easy definition, development and management of big data applications and services for analytics. The BigDataStack platform aims to provide a set of tools and services to simplify the development and management of big data applications, covering the full data pipeline. It provides valuable information about the data traffic in applications and bases infrastructure management decisions on data analytics of the system and applications running on it. With BigDataStack, we aim to help organizations enjoy the benefit of big data applications, reducing development efforts and increasing the performance of the applications at the same time.
Lessons learned in BigdataStack
For the development of BigDataStack, we have analysed the needs of big data applications and services, and we are currently working on the implementation of 3 use cases from the naval, insurance and retail industries. While doing so, we have learned some lessons about the barriers and challenges that big data projects involve.
- Requirements vary for different types of applications, stakeholders and organizations. For example, if a requirement is violated, in a predictive maintenance application for ships it might mean the ship could sink, for an insurance company this would mean losing money and in the retail case, one requirement violation might mean a page will load slowly.
- The applications’ performance largely depends on their deployment, but also on their current load. Most times, it is very hard to anticipate these things before the application is already functioning and because of this, compliance with their requirements is not guaranteed.
- Most organizations do not know what data they need to apply big data in their domain. This is crucial for GDPR since it states that we need to declare clearly what data is saved and what it will be used for. Data visualization can help to define the problem we are dealing with and how we can solve it. Data Visualisation is integrated in the BigDataStak holistic solution.
- Difficult to know the right amount of resources that Big Data applications will need in their final deployment. This is especially important for SMEs that cannot overprovision resources because they do not have as many resources as large companies do. Many scaling solutions that aim to help with this problem have become popular, like the ones offered by Amazon Web Services and Microsoft Azure, but still, with these auto-scaling solutions, the mapping between performance and requirements is not always correct and applications’ requirements can be violated. BigDataStack works on an auto-scaling solution.